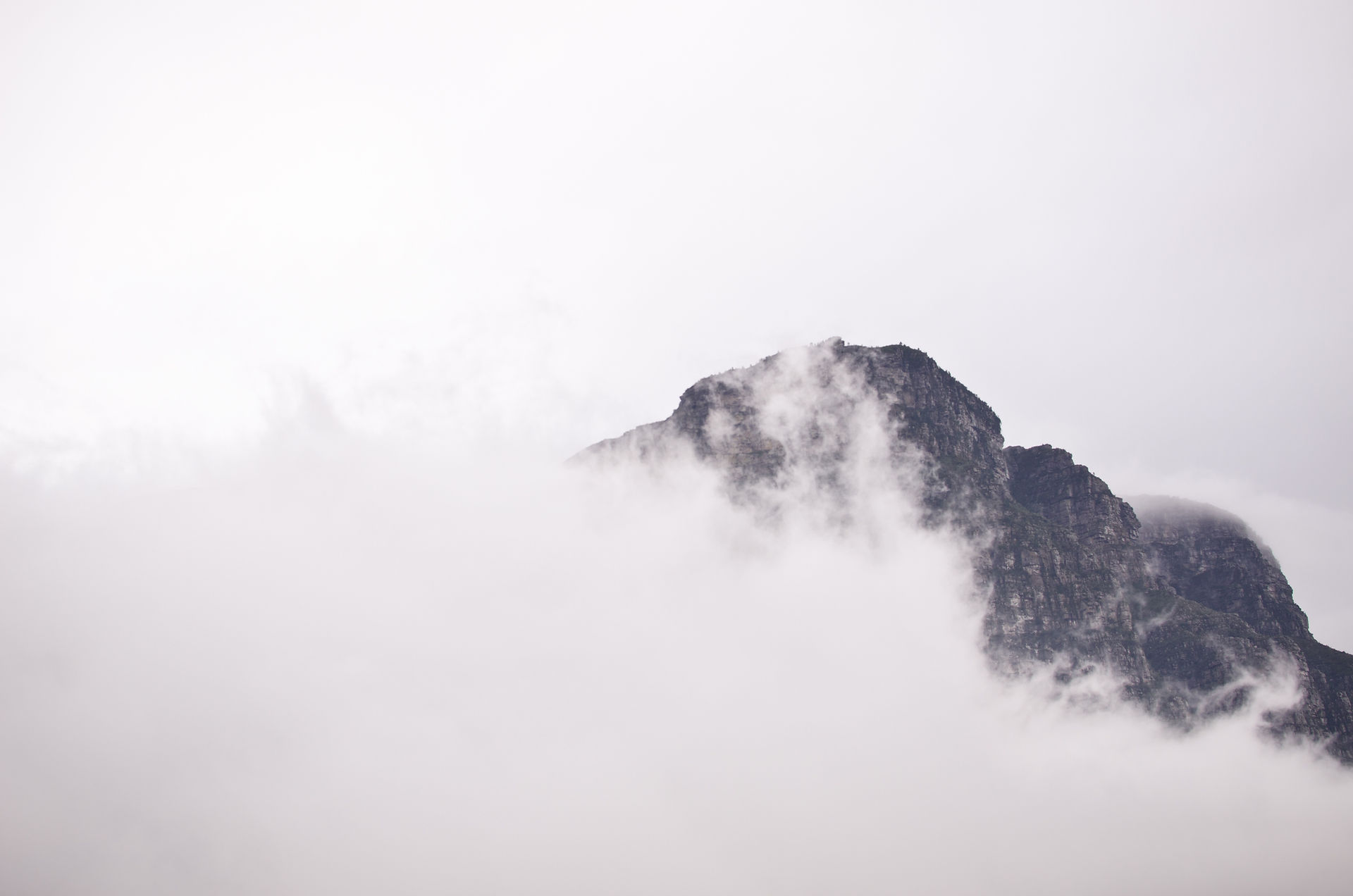
Sushil Raj Poudel , PhD
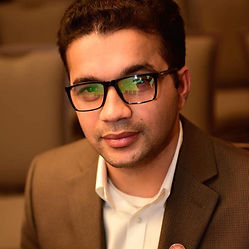
Dr. Sushil Raj Poudel is a highly accomplished professional with a distinguished career in industrial and systems engineering. He holds a PhD in Industrial & Systems Engineering from Mississippi State University, where he developed innovative models and algorithms to address complex issues in biomass supply chain networks under uncertainty.
Dr. Poudel currently serves as a Senior Operations Research Analyst at UPS, where he leads a team in building, prototyping, and deploying optimization solutions for optimal network planning. His role involves extensive research and application of advanced analytics, machine learning, and artificial intelligence to solve real-world supply chain problems.
In addition to his corporate experience, Dr. Poudel is an Adjunct Professor at Westcliff University, where he facilitates courses in Agribusiness Supply Chain Management for students at Kings College, Nepal. His academic contributions extend to his time as a Research & Teaching Assistant at Mississippi State University, where he developed and applied stochastic programming models, dynamic multi-modal transportation networks, and advanced solution algorithms.
Dr. Poudel's research has been widely recognized and published in reputable journals, and he has contributed a book chapter on bioenergy supply chains. His work has earned him several prestigious awards, including the Best PhD Student Award and the MSU Presidential Diversity Award. He has also been actively involved in professional organizations such as INFORMS and the Institute of Industrial Engineers.
​
MY LATEST RESEARCH
We have used a prediction model to predict the supply of cornstover and solved the two-stage stochastic problem to minimize the total expected system costs for biomass supply chain for co-firing.

Sample Average Approximation (SAA) has been used to circumvent the computational difficulties associated with large scenario stochastic programming problem. We further added the Progressive Hedging(PH) some heuristic approach and Rolling Horizon (RH) to enhance the computational efficiency of the algorithm. The comparison between the results of the proposed approaches shows that the addition of PH, heuristics and RH gradually increased the efficiency of the computation. For example : to solve 40 scenario problem with 5 replication CPLEX and PH went out of memory. PH with heuristics added was able to solve to it's optimality gap of 0.90\% in 9960 CPU times, while PH with heuristics and RH was able to solve to it's optimality gap of 0.00\% in 9900 CPU times.